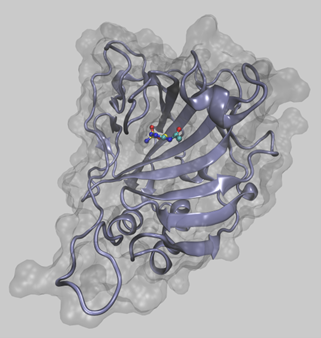
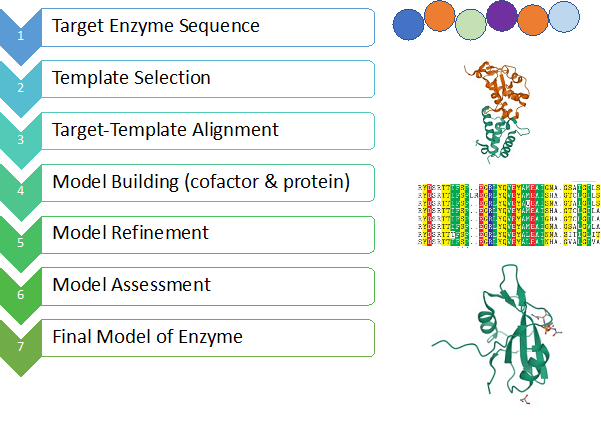
Introduction:
The complex interplay between protein structure and function is of immense interest in enzymology, where the 3D structure of the protein determines the activity of enzymes. Experimental methods of protein structure determination are high resolution in nature and often very expensive to obtain. Therefore, alternative approaches are in high demand. Computational methods are highly useful in modelling protein structures, however, there is a trade-off between accuracy and computational cost. Even with low computational cost, there exists plenty of methods to model protein structures. Such methods are highly useful in the research field of enzymology.
Recent developments in structural biology resulted in the characterization of massive number of protein structures, which in turn, result in the fortification of our understanding in the paradigm of structure-function relationship in the world of proteins. The field of enzymology, wherein the deeper understanding of the 3D structures of enzyme-substrate complex, transition state, and product is of paramount interest, is greatly benefitted from this advancement. This information is not only helpful for the deduction of molecular mechanisms behind the catalytic action of enzymes, it also helps immensely to design or engineer novel enzymes with improved outcomes.
Recent advances in structure determination methods, such as high throughput procedures in Nuclear Magnetic Resonance (NMR) spectroscopy and X-ray crystallography techniques have resulted in the deposition of huge number of protein structures in Protein Data Bank (PDB). However, this number is far less when compared the number of sequences being deposited in protein sequence database, owing to the inherent bottlenecks (time, cost, and complexity) associated with protein structure determination techniques. This resulted in a big gap between number of protein sequences and available protein structures. It is impossible to fill this gap by accelerating the experimental methods of protein structure determination alone. Therefore, there have been high demands in alternative approaches in acquiring structural information, including both experimental methods (such as low-resolution cryo-electron microscopy) as well as computational predictions (such as homology modelling).
Development of advanced computer components such as multi-core processors, graphical processing units, memory chips and solid-state hard disks has revolutionized the modern computers. This massive growth in the production of faster computers led to the development of computational approaches in every field, including biological and medicinal research. In structural biology, computers are increasingly used to predict the native structure of protein, simulation of their dynamical behavior and Quantitative Structure Activity Relationship (QSAR). All these methods have been successfully used in enzymology and drug design. In this blog, we review the computational methods for protein structure prediction, which find application in enzymology research.
Protein structure prediction method
Homology modelling or comparative modelling uses a known protein structure (template) information to predict the structure of target protein, which shares significant sequence similarity with the template.1 This method is based on the fact that proteins that have similar sequence are usually similar in structure and therefore they are functionally similar. This method is robust, and it often results in high quality structures of enzymes, which can be used to understand their mechanism of action.
First step in homology modelling is to look for a suitable template for the target sequence, typically using a sequence alignment tool such as BLAST (Figure 1). Template is a protein whose structure is already known and shares at least 40% sequence similarity with the target. The limit of 40% similarity is imposed to ensure the sequence similarity has evolutionary origin, rather than random matching of amino acids. After the identification of template protein, an alignment between target sequence and template structure is created, which defines the positions of target sequence on the template structure. Using the alignment information, a few models are generated, and these models are often subjected to extensive optimization. The models are then assessed, typically based on atomic contacts, packing quality, hydrogen bonding and burial of hydrophobic amino acids. The reliable models can be used to study their interaction with drug candidates.
In the cases where templates could not be identified or target-template sequence similarity falls below 30%, other modelling strategies (such as threading or ab initio protein modelling) could be used. However, these methods often produce low-resolution models. Although the low-resolution models are mostly used for functional annotations, their use in drug research is rather limited.
Recent trends
Much of the current research in protein structure prediction is focused on the development of new techniques to produce high quality models even if the target-template sequence similarity is significantly lower. It has been proposed by many that inclusion of multiple templates or multiple sequence alignment enhances the accuracy of the models.1,2 Further improvements in the alignment can be attained by the inclusion of secondary structure prediction data for the target sequence. This is very useful if the sequence similarity falls in twilight zone.
In addition, much of the research is devoted to the optimization protocols to refine protein structure models resulting from poor alignment (i.e., low sequence similarity).3 The optimization methods include simple energy minimization and extensive conformational sampling such as simulated annealing, Monte-Carlo search, and molecular dynamics (MD) simulation. Of these, MD simulation is of particular interest in molecular modelling community. MD simulation couples Newton’s equations of motion and classical force field potential energy functions to simulate the dynamical behavior of molecules at the atomistic level. Albeit the inaccuracy in the force field energy functions, this method has many success stories in biomolecular modelling. Many variants of MD simulation are employed in protein structure optimization such as replica exchange MD simulation (REMD), accelerated MD (aMD), multi-scale MD and steered MD. In many cases, a protein is modelled as individual fragments and the fragments are assembled, much like assembling a motorbike using individual components.
The performance of different modelling schemes are often evaluated in an annual meeting called “Critical Assessment of Structure Prediction (CASP)”. Once in two years, researchers are invited to submit their models for a set of proteins for which the experimental structures are not publicly available.4 The predicted models are assessed based on their agreement with the experimentally determined structures. The parameters used for the assessments are inter-residue contacts and distances, to obtain Global Distance Test (GDT_TS). Hence, GDT_TS is a multi-scale indicator (expressed in %) to measure the similarity between protein structures. The score was developed by Adam Zemla at Lawrence Livermore National Laboratory and originally implemented in the Local-Global Alignment (LGA) program. In general, template-based modeling methods have been very accurate if homologous proteins are available. However, in the absence of homologous structures, Artificial Intelligence/Machine-Learning approaches have been successful.
There are many online resources available for the end users to perform homology modeling. SWISSMODEL is a very intuitive web interface to perform homology modelling in different levels of difficulty. Modeller is a desktop application which offers a wide range of functionalities, including MD-based refinement of structures. I-TASSER is another web-based tool to model protein structures. I-TASSER uses replica exchange Monte Carlo conformational sampling to optimize protein structures. The accuracy of I-TASSER in modelling protein structures is evident from the CASP results, which show the tool is top ranked in several assessments. Rosetta suite of programs offer Denovo Structure Prediction, i.e., prediction of protein structure using the sequence as the only information to model. More recently, AlphaFold (an AI/ML based approach) has been reported to have predicted the structure for almost all the sequences in the sequence database, albeit with some limitations. This further opens a new avenue for protein structure prediction methodologies. In the recent CASP14 assessment, AlphaFold2 was the star performer which predicted both global fold similarity as well as local interaction details with great accuracy (TM-score > 0.85).
In recent years, there has been tremendous effort from researchers to improve the accuracy of the protein structure prediction. Such initiatives result in the reliable prediction of protein structures with minimal information from templates. Advances in computer hardware reduce the computational cost associated with the methodology and therefore the methods are available at everyone’s disposal. Accurate protein modelling, together with protein-ligand docking methods, can be very useful in computational enzymology research, as it reduces cost and time.5 At Quantumzyme, we successfully employ protein structure prediction as one of the tools for enzyme discovery and engineering research for the industrial and environmental applications and for the greater greener future.
For a related feed on protein structure prediction by AlphaFold, follow this link https://blog.quantumzyme.com/blog/?p=373
Reference:
- Li J, Cheng J. Scientific reports. 2016, 6:25687.
- Sokkar P, Mohandass S, Ramachandran M. Journal of molecular modeling. 2011, 17:1565-77.
- Kumar V, Meyer D, Lall N. J Proteomics Bioinform. 2017, 10:284-97.
- Moult J, Fidelis K, Kryshtafovych A, Schwede T, Tramontano A. Proteins: Structure, Function, and Bioinformatics. 2018, 86:7-15.
- Pandian Sokkar. Chettinad Health City Medical Journal 2020; 9: 217 - 219