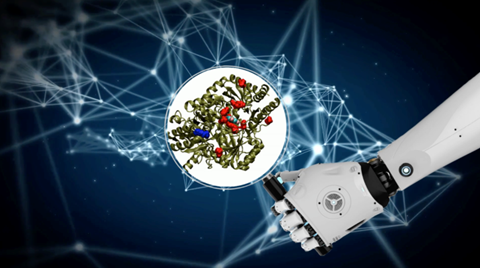
Enzyme engineering is the process of improving the efficiency of an existing enzyme for enhanced catalytic activity or stability by altering its amino acid sequence. This is performed to overcome the limitations of native enzymes for industrial biocatalytic applications. Traditionally, enzyme engineering is carried out either by random mutagenesis experiments or by rational structure-based computational approaches. Recently, computing technologies such as Artificial Intelligence (AI) and Machine Learning (ML) are being explored to engineer enzymes.
AI and ML are the part of next gen computing technology that correlates with each other. Although these are two related technologies and sometimes used as a synonym for each other, both are two different terms.
Broadly, AI and ML can be differentiated as:
AI is a bigger concept that creates intelligent machines that can simulate human thinking and behavior, whereas, machine learning is a part of AI that allows machines to learn from data without the need for programming explicitly.
At Quantumzyme, we work with both AI and ML to enhance the capabilities of a given enzyme by enabling the possibility of seeing several hundred mutations in the least amount of time. The first step to building efficient AI model is to get a robust ML algorithm in place and this is dependent on the quality of the data set. The enzyme engineering community has recently started focusing on curating digital data. But it is still in its nascent stage and it will only get better. So, what kind of data do we need for AI/ML?
Larger accumulation of data has proven to be very useful for building AI / ML models, as these algorithms strive on understanding from more and more relevant data. Current data available on various databases are good for volume but mostly focused on protein sequence. They lack the details regarding the protein structure, enzyme kinetics & performance and reaction information.
Most databases are not well structured, which poses problems in data retrieval and hence it cannot be directly used for machine learning models. One would need to clean and preprocess the gathered data prior to the AI/ML computing. Many a times, it is not possible to extract necessary information from databases, as the data may not be present in usable formats, such as images or data without definite structure. The databases acquired from combining data accumulated from various sources may provide enough quantities of data, but the problem arises with respect to the data consistency and comparability.
The ML umbrella also encompasses another term, deep learning. Deep learning models are networks of large size and complexity that are able to model difficult data distributions such as data of high dimensionality. These models extract useful patterns directly from the raw data without the need for manually created features or any human intervention. However, due to the large number of parameters in deep learning models, exhaustive training data sets are required for model training/learning. With more experimental data and more computational power available, ML efforts in the field of enzymology is growing and will grow, including the creation of new databases for training, data preprocessing and the ethical concerns related to creating new biomolecules with unintended characteristics.
There is a need for efficient technology solution to ensure positive outcomes with great work being done in this field. This technology should be able to cut down tasks by implementing automation and help the investigator to understand the problem better by giving more insights, visual illustrations of complex concepts and provide intelligent predictions. Following is Quantumzyme’s QZyme WorkbenchTM modules which makes use of AI/ML in its modules:
- Qzyme Pilot™: is to help search for relevant info over the web, in this a recommendation engine plays an important role, which is able to crawl over webpages and finding associations between relevant information and placing all of them in one place.[PS1] [RS2]
- Qzyme Modeller™: modelling is all about developing a 3D model of the enzyme in absence of accurate or limited information, and it becomes very challenging when information available is very less. ML models can be trained with relevant data to predict such structures with an increased accuracy.
- Qzyme Catmec™: This includes docking, simulation, binding affinity calculation, free energy calculations and etc. which leans on data analytics and deep learning.
- Qzyme Hotspot™: Dealing with discovering the key functional residues in substrate binding, ML plays a vital role in getting more insights about the structure and information about finding out positions for mutations.
Qzyme Designer™: With the QZyme Hotspot picking the specific positions through ML, the QZyme Designer uses the ML algorithms to predict the best mutations at those positions to get results of better efficiency, or mutations that can yield better results.
In conclusion, AI/ML is extremely critical to leap forward and achieve success in Enzyme Engineering. In the past decade there has not been any specific focus on this but the turn of this decade has pushed harder on the need for faster solutions for newer and higher efficient enzymes. Within Quantumzyme, AI/ML has been proven to be one of the most successful approaches alongside the workhorse of computational chemistry techniques pipelined in QZyme WorkbenchTM.