
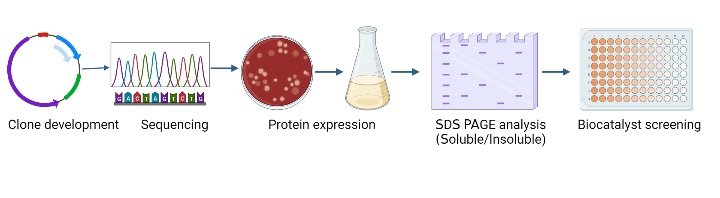
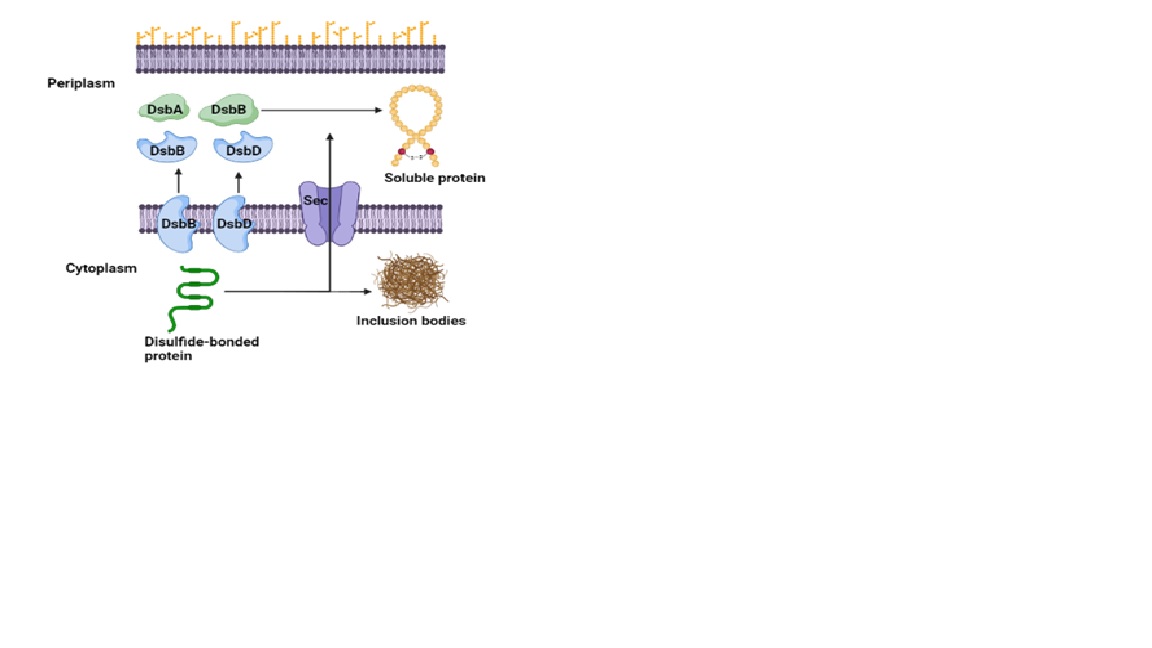
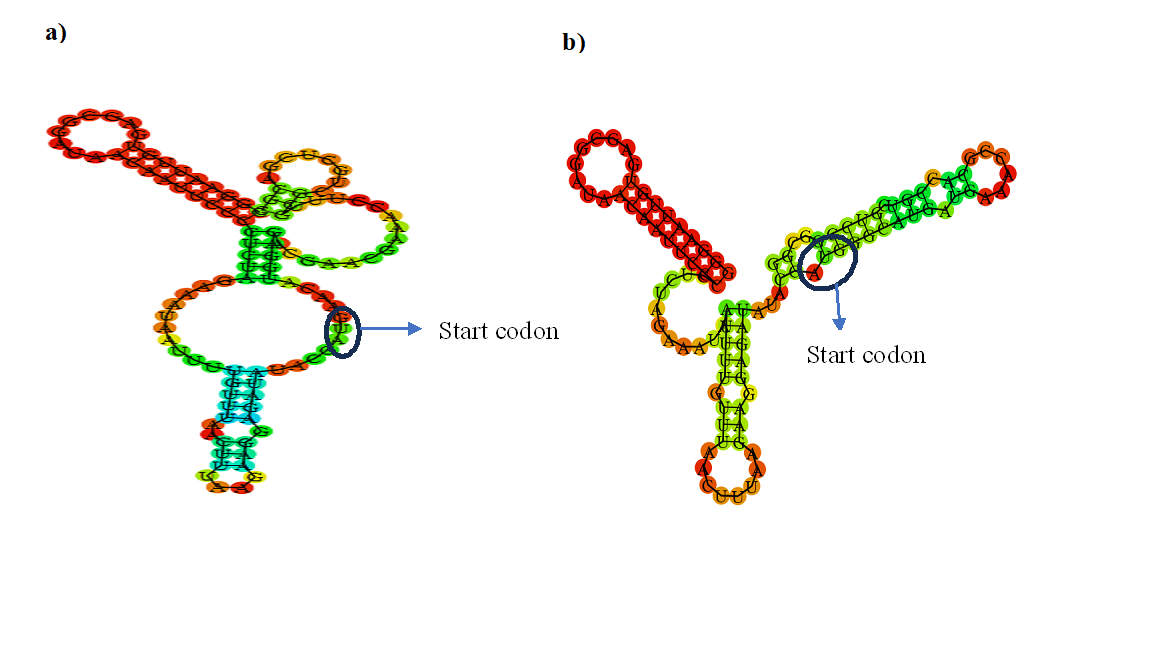
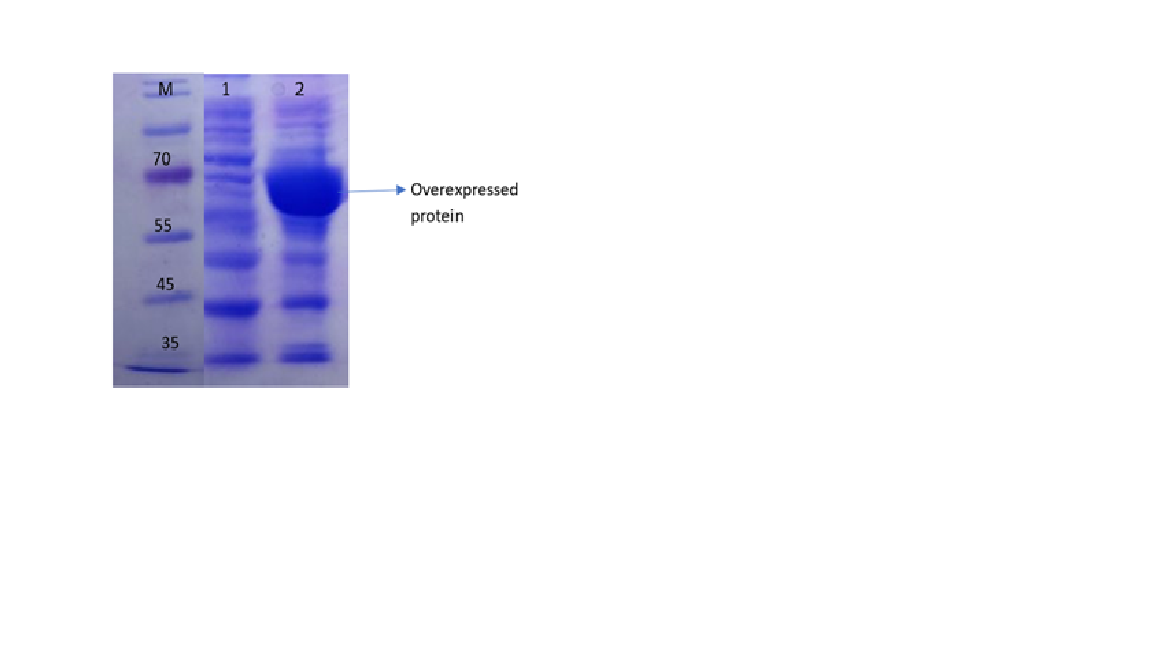
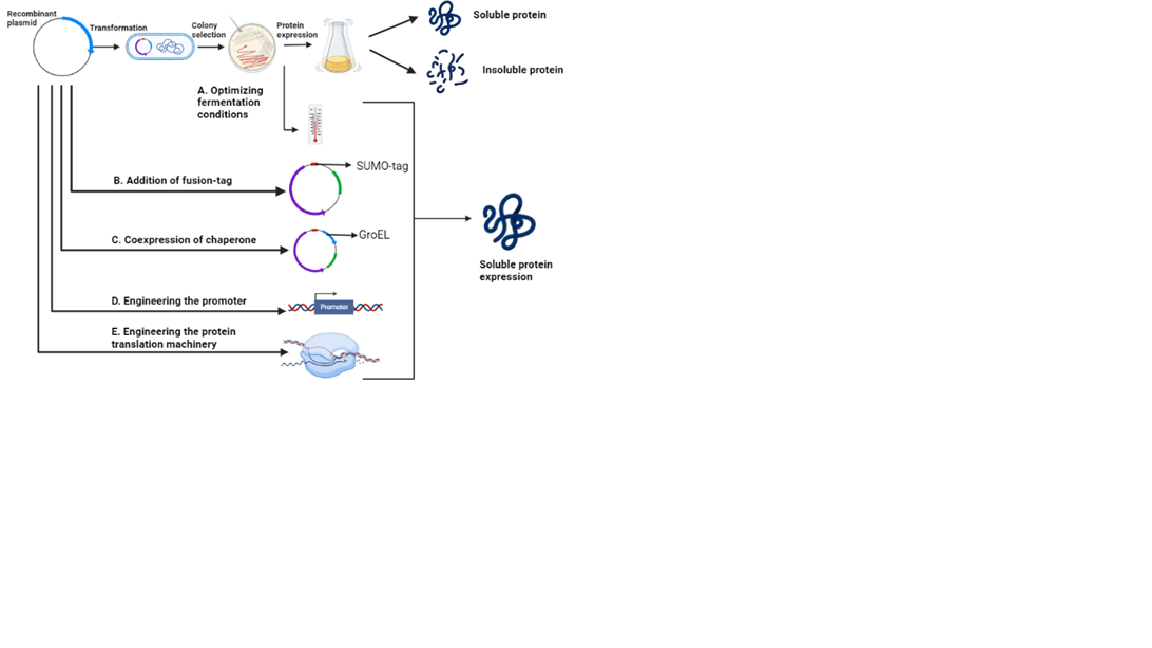
Screening of biocatalysts:
In the past few years, the number of protein sequences in the gene database has increased exponentially. Almost 50% of the deposited sequences are uncharacterized and hypothetical proteins with incorrect functional annotations. The protein sequences extracted from the database were recombinantly expressed in a suitable expression host. Escherichia coli (E. Coli) is the most preferred expression host for the expression of biocatalysts for various reasons (i) well-known genetics and physiology, (ii) availability of compatible molecular tools, (iii) fast growth rate and inexpensive media, (iv) Genetically regarded as safe, (v) economically attractive. However, recombinant biocatalysts expression in E. coli is not always successful since most of them are expressed as inclusion bodies (IB) or result in low expression or cell death due to protein toxicity (Figure 1). IBs are typically insoluble and biologically inactive proteins. The expression of proteins in insoluble form is undesirable, even though they could be refolded in vitro to attain catalytically active protein. Furthermore, the recovery of soluble protein from IB is very low and time-consuming due to labor intensive processes such as washing, solubilization and refolding and usage of harsh chemicals such as urea and guanidine hydrochloride. Hence, the success rate in obtaining the soluble biocatalysts requires an efficient screening method that considers a wide array of factors.
Figure 1: Overview of recombinant biocatalyst expression and screening.
This challenge can be overcome by using bioinformatic tools which can help in screening of potential proteins for recombinant expression. Bioinformatics tools predict the gene expression and soluble protein production by considering information including the codon usage, transcription, and translation efficiency. Below are some of the predictions to be considered while screening for the potential genes.
1. Disulfide bond prediction:
The recombinant proteins can either be expressed in the cytoplasm or periplasm of E. coli. Most of the proteins were expressed in the cytoplasm. However, the lack of an oxidative environment makes it difficult to express proteins containing disulfide bonds. The disulfide bonds are synthesized in the oxidizing environment such as endoplasmic reticulum in eukaryotes and the periplasm in prokaryotes. The disulfide bonds are synthesized by sulfhydryl oxidase and disulfide bond isomerase. The cytoplasmic environment of E. coli is more reducing due to higher negative redox potential, and contains thioredoxin reductase and the glutaredoxin reductase enzymes, which are required for the reduction of disulfide bonds. Hence, the disulfide-bonded proteins must be targeted to the periplasm for soluble protein production (Figure 2). Alternatively, engineered E. coli strains such as SHuffle, Origami, Rosetta Origami, CyDisCo allows the expression of disulfide-bonded proteins in the cytoplasm. However, the success rate of recombinant expression in the engineered E. coli strains is low due to unfavourable genetic background.
Figure 2: Overview of recombinant expression of disulfide-bonded proteins in E. coli. The secretion pathway translocates the proteins to the periplasmic environment, where the Dsb proteins facilitate the disulfide bond formation.
The presence of disulfide bonds can be predicted by using the protein sequence or structure as input. DIpro (SCRATCH-protein predictor), a cysteine disulfide bond predictor can be used to predict the presence of disulphide bonds in protein and the number of disulfide bonds (Table 1). The data from Table 1 shows the importance of predicting disulphide bonds prior to protein expression in lab. This will help to identify the suitable vector for cloning, expression host, and localization of proteins.
At Quantumzyme, we make use of disulphide bond prediction as part of Enzyme discovery projects to evaluate the suitability of the enzymes for recombinant overexpression in lab. A comparison of disulphide bond prediction and lab validation performed for Quantumzyme’s (QZ) ketoreductase and hydratases is given below:
Table 1: Disulphide bond prediction and lab validation for QZ enzymes
Enzyme class | Enxzyme code | Disulfide bonds predicted | Recombinant expression localization | Protein expression as evaluated by SDS-PAGE |
Ketoreductase | KRED-001 | No | Cytoplasm | Soluble |
Ketoreductase | KRED-002 | No | Cytoplasm | Soluble |
Ketoreductase | KRED-003 | No | Cytoplasm | Soluble |
Linalool hydratase | LHD-001 | Yes (2 disulfide bonds) | Cytoplasm | Inoluble |
Linalool hydratase | LHD-002 | Yes (2 disulfide bonds) | Cytoplasm | Inoluble |
2. mRNA secondary structure prediction:
mRNA secondary structure is one of the major factors influencing protein expression in E. coli. Secondary structures in the ribosomal binding site (RBS) of mRNA can reduce the translational efficiency by competitive inhibition, where the cis mRNA competes with 30S subunit and binds to the RBS forming inactive complex. The secondary structures of mRNA at the translation initiation region (TIR) plays an important role in the production of recombinant proteins. The stability of structures at the RBS determines the efficiency of the translation. Based on the quantitative correlation between the thermodynamic stability of RBS and translation efficiency, secondary structures with minimum free energy (MFE) less than -6 kcal/mol do not affect the translation efficiency. mRNA secondary structures with open conformation at the start codon (AUG) are easily accessible by the ribosomes thus enabling efficient translation (Figure 3). Several computational tools that predict the mRNA secondary structures with or without pseudoknots are available, including DMfold, Mfold, RNAsoft, UFold, RNA-SSPT, RNAfold, NUPACK, RNAStructure, pknotsRG, CONTRAfold, CycleFold, LearnToFold, MXfold, and SPOT-RNA. The predictions of mRNA secondary structures and MFE by computational methods is a key to the successful expression of heterologous proteins.
Figure 3: mRNA secondary structure prediction for a) QZ-ketoreductase, b) QZ- linalool hydratase gene cloned in pET28 a (+) vector. mRNA secondary structure of ketoreductase has open RBS, whereas the linalool hydratase has closed RBS within the stem loop. The expression of the ketoreductase and linalool hydratase in E. coli yielded high and medium overexpression, respectively.
3. Protein solubility prediction
Protein solubility prediction is an active area of research. Protein solubility depends on intrinsic factors such as protein sequence and structure, molecular weight, amino acid composition, hydrophobicity, aromaticity, isoelectric point, structural propensities, and polarity residues on the surface, as well as extrinsic factors such as ionic strength, temperature, and pH. Many solubility prediction tools were developed considering these features. Some of the existing protein solubility prediction tools are PROSO II, SOLpro, ccSOL, PROSO, Soluprot, DSResSol, SVM-based, SI-based, Recombinant Protein Solubility Prediction, ProS, ESPRESSO, Scoring Card Method, CamSol, Protein-Sol, DeepSol, and PaRSnIP. The solubility prediction tool classifies the protein as either soluble or insoluble based on the solubility score considering the features such as hydrophobicity, lysine to arginine ratio and protein charge and size. For example, Protein-Sol, a sequence-based prediction tool, calculates the solubility score based on the amino acid sequence. Proteins with a scaled solubility score greater than 0.45 are highly soluble and scores less than 0.45 are predicted to be less soluble (Table 2). The data from Table 2 shows that the bioinformatic prediction is correlating with the lab studies. This shows the importance of predicting the protein solubility and the trial-and-error procedure of recombinant expression can be avoided by identifying the soluble protein candidates.
Table 2: Protein solubility prediction and lab validation for QZ enzymes
Enzyme class | Enxzyme code | Protein-Sol prediction score | Recombinant expression as evaluated by SDS-PAGE |
Ketoreductase | KRED-001 | 0.592 | Soluble |
Ketoreductase | KRED-002 | 0.569 | Soluble |
Ketoreductase | KRED-003 | 0.583 | Soluble |
Linalool hydratase | HD-001 | 0.162 | Inoluble |
Linalool hydratase | HD-002 | 0.102 | Inoluble |
4. Codon optimization
The level of recombinant protein expression in microorganisms is affected by codon usage bias. Rare codons can reduce the translation rate and increase the translational errors. Codon optimization is therefore an important aspect of gene synthesis since it improves the expression of synthetic genes in heterologous host organisms. Codon optimization is the process of converting the nucleotide sequence of one species into the nucleotide sequence of another species. There are two strategies for codon optimization. The first one is one amino acid-one codon, which allocates the most abundant codon of the host or a set of selected genes to a given amino acid in the target sequence. The second strategy, codon randomization, is based on the frequency distribution of the codons in a genome or a subset of highly expressed genes and uses translational tables. Each codon has a weight assigned to it. Several web-based codon optimization tools are available such as DNAWorks, Synthetic Gene Designer, COOL, Gene Designer 2.0, and OPTIMIZER. Gensmart, a codon optimization tool developed by Genscript considers more than 200 factors involved in gene expression including GC content, codon usage, RNase splicing sites, cis-acting mRNA destabilizing motif, and codon adaptation index (CAI). CAI value of 0.8-1.0 is considered ideal. The lower the CAI value, the higher the chance of less recombinant protein expression (Table 3 and Figure 4). The data shows the importance of codon optimization to achieve a high protein overexpression in E. coli.
At Quantumzyme, we make use of Genscript services (Gensmart tool) as part of Enzyme discovery and engineering projects to codon optimize the selected genes for recombinant expression in E. coli. Codon optimization and lab validation performed for QZ enzymes by Gensmart tool is given below:
Table 3: Codon optimization and lab validation for QZ enzymes
Enzyme class | Enxzyme code | CAI value | Codon-optimized gene expression (++: High, +: Medium) as evaluated by SDS-PAGE |
Ketoreductase | KRED-001 | 0.96 | ++ |
Ketoreductase | KRED-002 | 0.96 | ++ |
Ketoreductase | KRED-003 | 0.98 | ++ |
Linalool hydratase | LDH-001 | 0.97 | + |
Linalool hydratase | LDH-002 | 0.95 | ++ |
Figure 4: SDS-PAGE analysis of codon-optimized QZ enzyme expressed in E. coli. Lane 1: Protein marker, Lane 2: QZ uninduced sample, and Lane 3: QZ induced sample.
5. Protein folding rate prediction
The fate of the protein to be either soluble or insoluble depends upon the folding mechanism. Proteins have different rates of folding. Protein folding occurs in a hierarchical order to produce stable native state. The failure to fold to its native state results in misfolded or aggregate proteins. Kinetic order and rate constant are the important factors to understand the protein folding mechanism. Kinetic order, which determines whether the protein reaches its native structure through one or multiple intermediates; rate constant, which varies from nanoseconds to hour depending on the protein length. Experimental determination of folding kinetics and rate constant is generally time-consuming and labour-intensive process. Hence, bioinformatic tools have been developed as an alternative. Predicting the protein folding rate from the amino acid sequence has become a popular field of research. Some of the existing tools are SFoldRate, FOLD-RATE, Pred-PFR, FoldRate, K-Fold, PRORATE, Folding RaCe. The protein folding rate data will be helpful to express the recombinant proteins in a suitable expression vector and promoter. A protein with a faster folding rate can be expressed using a strong promoter in a medium or high copy number plasmid, whereas a protein with slow folding rate must be expressed using a weak promoter in a low copy number plasmid.
In vitro strategies to improve the soluble biocatalysts production:
1. Optimization of protein synthesis rate
For soluble protein expression, the recombinant E. coli cells should be grown at 37⁰C until they reach mid-to-late log phase, after the cells should be induced between 15 to 25⁰C. Lowering the temperature decreases the protein aggregation within the polypeptides.
2. Co-expression of molecular chaperones
Co-expression of chaperones such as DnaK, DnaJ, GrpE, GroEL and GroES would prevent aggregation of mis-folded proteins by preventing improper interactions within and between other polypeptides. The chaperones can be co-expressed with the gene of interest in a single plasmid or as two different plasmids to improve the solubility (Figure 5).
3. Fusion protein technology
Fusion proteins or short peptide tags are used as an alternative to chaperone co-expression for improving the solubility of recombinant proteins. The fusion tags can be genetically linked either at N-or C-terminal with the target protein to improve the solubility. Fusion tags improves the solubility by forming a micelle like structures and prevent aggregation.
Figure 5: Strategies to improve the soluble protein production.
4. Engineering the protein translation machinery
A spacer length of 7-10 nucleotide length between RBS and the translation initiation codon (AUG) in the expression vector has proven to be effective for soluble expression in E. coli. Alternatively, introducing slow-translating regions in the gene during the codon optimization process improves the protein solubility.
In conclusion, the bioinformatic tools would eliminate the trial-and-error procedure of testing various biocatalysts in the laboratory. Incorporation of bioinformatic tools would improve the production yield and reduce the production cost in industries. The production yield can further be improved by employing the molecular strategies to the proteins screened through bioinformatic approaches.
References:
- Khurana, Sameer, et al. "DeepSol: a deep learning framework for sequence-based protein solubility prediction." Bioinformatics 34.15 (2018): 2605-2613.
- Gromiha, M. Michael, A. Mary Thangakani, and Samuel Selvaraj. "FOLD-RATE: prediction of protein folding rates from amino acid sequence." Nucleic acids research 34. Suppl 2 (2006): W70-W74.
- Arya, Ranjana, et al. "Optimization of culture parameters and novel strategies to improve protein solubility." Insoluble proteins: methods and protocols (2015): 45-63.
- Manta, Bruno, Dana Boyd, and Mehmet Berkmen. "Disulfide bond formation in the periplasm of Escherichia coli." EcoSal Plus 8.2 (2019).